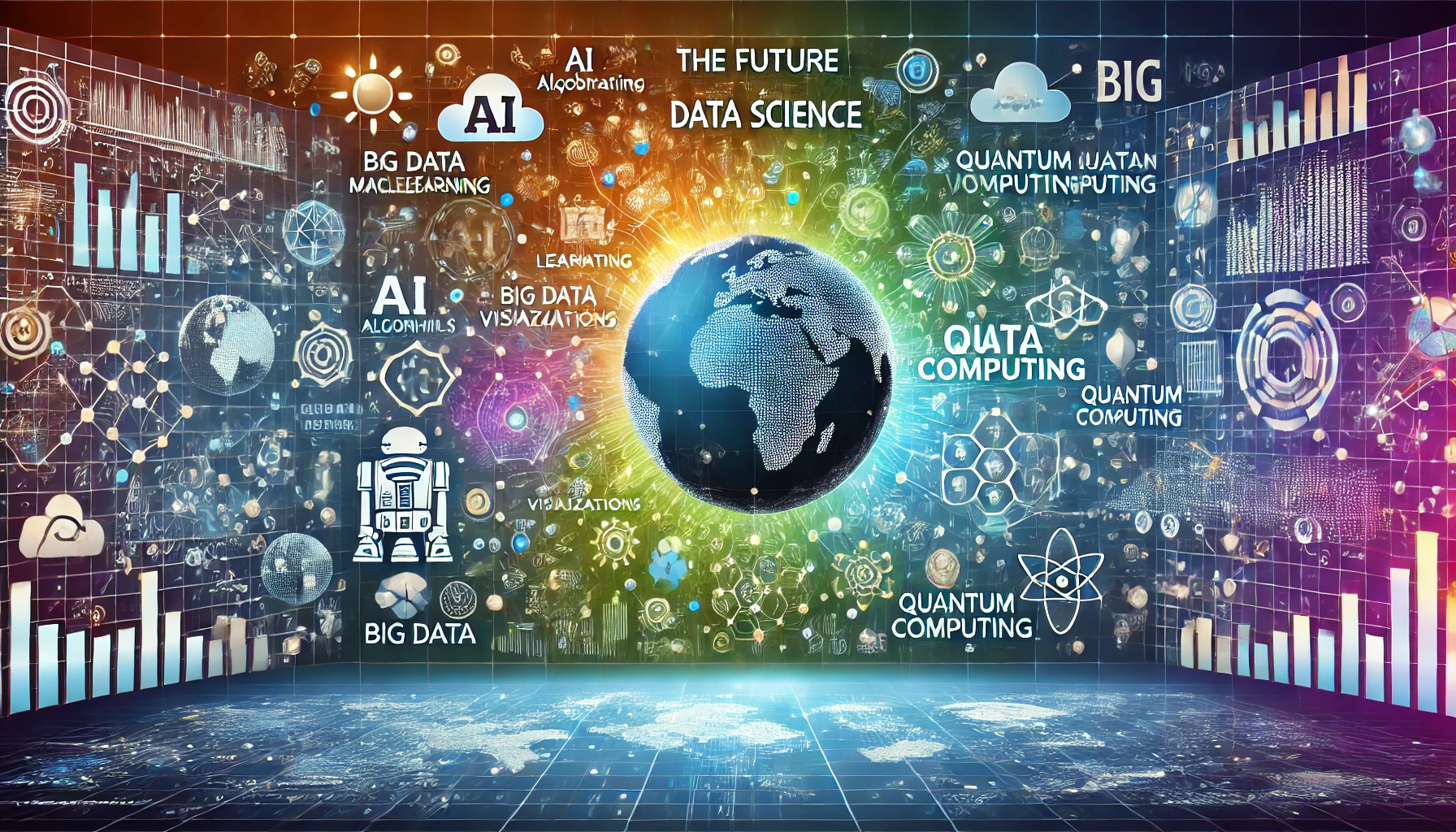
The Future of Data Science
Introduction
Data science has become a critical force driving innovation across industries, from healthcare to finance, manufacturing, and beyond. Its role in analyzing massive data sets, predicting outcomes, and aiding decision-making has made it indispensable in today’s digital age. As data continues to grow exponentially in volume and complexity, the field of data science is set to evolve, driven by technological advancements, increased automation, and the integration of AI and machine learning. The future of data science holds incredible potential, with new technologies, ethical considerations, and novel applications waiting to emerge.
In this article, we’ll explore the trends shaping the future of data science, the technologies driving its growth, and the societal impacts we can expect in the coming years.
The Rapid Growth of Data Science
The Increasing Importance of Big Data
The proliferation of digital devices and online platforms has resulted in the creation of enormous amounts of data. This phenomenon, known as Big Data, is at the core of modern data science. Organizations are increasingly relying on data-driven insights to improve decision-making, enhance customer experiences, and optimize operations. The sheer scale of data available today is unprecedented, and managing this vast information is both a challenge and an opportunity for data scientists.
Big Data is expected to grow further, fueled by the rise of the Internet of Things (IoT), social media, and cloud-based technologies. Data scientists must continue refining their methods to extract meaningful patterns from these large, unstructured data sets.
Evolution of Data Analytics Tools
As the volume of data grows, so does the sophistication of data analytics tools. Over the last decade, there’s been significant progress in the development of tools and platforms that allow businesses to process, analyze, and visualize data more efficiently. Platforms like Apache Hadoop, Spark, and cloud-based tools such as Google BigQuery and Amazon Redshift have become essential for handling large-scale data analytics.
In the future, we can expect these tools to become even more user-friendly and powerful. Advancements in natural language processing and AI will make it easier for non-technical users to interact with data through simplified interfaces, leading to the democratization of data science across industries.
Emerging Technologies Shaping Data Science
Artificial Intelligence and Machine Learning
One of the most significant influences on the future of data science is artificial intelligence (AI) and machine learning (ML). These technologies enable machines to learn from data and improve their performance without explicit programming. As AI and ML algorithms become more sophisticated, they are becoming critical components in data science workflows. From predictive analytics to recommendation systems, AI and ML models are transforming the way we interpret and use data.
In the future, AI-driven data science will likely become more autonomous, with less human intervention required in model building and decision-making. Automated machine learning (AutoML) tools are already allowing businesses to build and deploy models with minimal coding expertise. This trend is expected to continue, enabling faster and more accurate insights.
Natural Language Processing (NLP) and Speech Recognition
Natural Language Processing (NLP) and speech recognition technologies are also playing a significant role in shaping the future of data science. NLP enables computers to understand, interpret, and generate human language, making it a crucial tool in analyzing unstructured text data from sources such as emails, social media, and documents. As these technologies evolve, we will see more sophisticated applications, including real-time sentiment analysis and conversational AI systems.
Speech recognition technologies, driven by advancements in deep learning, are becoming more accurate and accessible. Their integration into data science platforms will allow businesses to analyze voice data and extract valuable insights from customer service interactions, telemedicine consultations, and more.
Internet of Things (IoT) and Sensor Data
The rise of IoT is generating vast amounts of sensor data from devices such as smart home appliances, industrial machinery, and wearable health trackers. As these devices become more prevalent, data scientists will need to develop methods to handle and analyze this continuous stream of information.
IoT data is often time-sensitive, requiring real-time processing to deliver actionable insights. This is where technologies like edge computing, which processes data closer to its source, will come into play. Data science will need to adapt to manage the complexities of IoT data and unlock its full potential for industries such as logistics, healthcare, and smart cities.
The Role of Automation in Data Science
Automated Machine Learning (AutoML)
One of the most groundbreaking advancements in data science is the rise of automated machine learning (AutoML). Traditionally, building machine learning models required extensive expertise in data preprocessing, feature selection, and hyperparameter tuning. AutoML tools simplify this process by automating these complex tasks, enabling organizations to create high-quality models with minimal manual intervention.
Platforms like Google AutoML and Microsoft Azure Machine Learning are already making it easier for businesses to harness the power of machine learning without needing a team of data scientists. In the future, AutoML is expected to continue evolving, allowing for more sophisticated models to be deployed faster and more accurately. This automation will not only accelerate the development cycle but also enable smaller organizations to adopt data science without the need for a large technical team.
Data Preprocessing and Automation Tools
Data preprocessing is often the most time-consuming step in data science, involving cleaning, transforming, and preparing raw data for analysis. Automation tools that handle data cleansing, feature engineering, and anomaly detection are rapidly becoming an essential part of the data science toolkit. These tools can save countless hours by identifying and correcting issues such as missing data, outliers, and inconsistent formats.
Looking ahead, we can expect further improvements in data preprocessing automation, reducing the need for manual intervention and allowing data scientists to focus more on model building and interpretation. As automation in data science increases, the role of human experts will shift towards higher-level tasks, such as defining business goals and interpreting results.
Quantum Computing: A Game-Changer for Data Science
Introduction to Quantum Computing
Quantum computing is a revolutionary technology that has the potential to transform data science in ways that are currently unimaginable. Unlike classical computers, which process data using binary bits (0s and 1s), quantum computers use quantum bits or qubits, which can represent both 0 and 1 simultaneously, thanks to a property known as superposition. This allows quantum computers to perform complex calculations exponentially faster than classical machines.
While still in its early stages, quantum computing is expected to revolutionize data processing, enabling the analysis of vast datasets that are beyond the reach of today’s computers. This will have a profound impact on industries like finance, cryptography, drug discovery, and logistics, where massive amounts of data need to be processed in real-time.
Impact on Data Processing and Model Building
The implications of quantum computing for data science are vast. Quantum algorithms will be able to solve problems that classical algorithms struggle with, such as optimization and complex simulations. In particular, machine learning models, which rely on extensive computations, could see unprecedented improvements in both speed and accuracy.
While fully functional quantum computers are still years away from mainstream use, companies like IBM, Google, and Microsoft are making significant strides in quantum research. As this technology matures, data scientists will need to learn how to leverage quantum algorithms to unlock new possibilities in data analysis and machine learning.
Ethical Considerations and Responsible Data Science
Privacy Concerns and Data Protection
As data science becomes more embedded in our daily lives, ethical concerns around privacy and data protection are growing. The collection and use of personal data raise serious questions about consent, security, and transparency. In recent years, regulations like the General Data Protection Regulation (GDPR) and the California Consumer Privacy Act (CCPA) have been implemented to safeguard individuals’ privacy rights.
Going forward, data scientists must ensure that their practices align with these regulations and that ethical principles are integrated into their workflows. This includes securing sensitive data, anonymizing personal information, and being transparent about data collection methods. As data breaches and misuse of information continue to make headlines, the need for responsible data science practices will only become more critical.
Bias in Algorithms and Fair AI
Another significant ethical challenge in data science is the issue of bias in algorithms. Machine learning models are trained on historical data, which may contain biases related to race, gender, and socioeconomic status. When these biases are embedded in the algorithms, they can lead to discriminatory outcomes, such as biased hiring decisions or unequal access to financial services.
Addressing bias in algorithms is essential for ensuring fairness and inclusivity in AI systems. Data scientists must actively work to detect and mitigate biases in their models by diversifying datasets, using fairness metrics, and regularly auditing their models for unintended outcomes. As AI continues to shape the future of society, ethical data science practices will be crucial for ensuring that technology benefits everyone, not just a select few.
Data Science in Healthcare
Predictive Analytics in Medical Research
Data science has already made significant strides in the healthcare industry, particularly in predictive analytics. By analyzing vast amounts of clinical data, data scientists can predict disease outbreaks, patient outcomes, and treatment success rates with remarkable accuracy. This ability to forecast health trends is revolutionizing medical research, enabling healthcare providers to proactively address patient needs and improve outcomes.
In the future, predictive analytics will continue to play a crucial role in personalized medicine, where treatments are tailored to the individual based on their genetic makeup, lifestyle, and medical history. This shift towards personalized care, powered by data, has the potential to drastically improve patient outcomes while reducing healthcare costs.
The Role of Data in Personalized Medicine
Personalized medicine is one of the most exciting frontiers in modern healthcare, and data science is at its core. By analyzing genetic data, environmental factors, and patient history, data scientists can develop individualized treatment plans that are far more effective than one-size-fits-all approaches. Machine learning algorithms are already being used to identify patterns in patient data that were previously undetectable, leading to more accurate diagnoses and tailored therapies.
As data collection technologies, such as wearable devices and IoT sensors, continue to evolve, the amount of health-related data available will increase exponentially. The ability to process and analyze this data will allow for more precise medical interventions, potentially leading to breakthroughs in the treatment of chronic diseases, cancer, and more.
The Rise of Edge Computing
What is Edge Computing?
Edge computing is an emerging technology that brings data processing closer to the source of the data itself. Instead of sending data to centralized cloud servers for processing, edge computing performs the computations at the "edge" of the network, on devices like smartphones, sensors, and IoT devices. This approach reduces latency and bandwidth usage, making real-time data processing more efficient.
As IoT devices proliferate, generating vast amounts of data, edge computing will become increasingly vital for data science. Real-time analytics will benefit from the reduced lag time, particularly in sectors such as healthcare, manufacturing, and smart cities, where immediate data insights are critical for decision-making.
How Edge Computing Improves Real-Time Data Analytics
The need for real-time analytics is growing rapidly, especially in industries like finance, healthcare, and logistics, where decisions must be made instantly based on the latest data. Edge computing offers a solution to this challenge by processing data locally, reducing the time it takes to derive actionable insights. This is particularly beneficial for IoT applications, such as autonomous vehicles, where even a few milliseconds of delay can have serious consequences.
As edge computing technology continues to mature, its integration with data science will enable new applications and innovations. Data scientists will be able to deploy models that operate directly on edge devices, allowing for faster, more efficient data processing and analysis.
The Growing Importance of Real-Time Data Processing
Real-Time Analytics in Finance and Retail
Real-time data processing is transforming industries that rely on quick decision-making, such as finance and retail. In finance, real-time data analytics enables faster and more accurate risk assessments, fraud detection, and algorithmic trading. Financial institutions can make immediate decisions based on market movements, reducing risks and increasing profitability.
In retail, real-time analytics allows businesses to optimize inventory management, personalize customer experiences, and improve supply chain operations. By analyzing data as it's generated, retailers can respond to changing consumer preferences and demand patterns, creating a more dynamic and responsive business model.
Innovations in Streaming Data Technologies
Streaming data technologies, such as Apache Kafka, Amazon Kinesis, and Apache Flink, are making it easier for organizations to analyze data in real time. These platforms enable continuous data ingestion and processing, allowing businesses to react to events as they happen. As these technologies evolve, we can expect more efficient and scalable systems that can handle the massive amounts of streaming data generated by IoT devices, social media, and financial markets.
The future of data science will increasingly rely on real-time data processing, particularly as industries seek to gain competitive advantages through faster insights and more dynamic decision-making processes.
The Integration of Data Science with Blockchain
Blockchain's Role in Securing Data
Blockchain technology, originally developed for cryptocurrency, is now making waves in data science due to its potential for securing data. Blockchain offers a decentralized and tamper-resistant system that can ensure the integrity and traceability of data. In a world where data security is paramount, especially with increasing concerns over privacy breaches, blockchain provides a solution for verifying and safeguarding large datasets.
By using blockchain, organizations can ensure that data has not been altered or tampered with, offering an additional layer of trust. In fields like healthcare, supply chain management, and finance, where data accuracy and security are critical, blockchain could be the key to building more robust and transparent systems.
Distributed Ledger Technology for Data Management
Distributed ledger technology (DLT), the backbone of blockchain, allows multiple participants to maintain and share the same data records securely. For data science, this creates opportunities for collaborative data sharing without the risks associated with a single point of failure or centralization.
In the future, DLT could facilitate the secure exchange of large datasets between organizations, enabling more collaborative research efforts and breaking down data silos. This would empower industries to analyze vast amounts of distributed data without compromising security or privacy, accelerating innovation and creating new opportunities for data-driven insights.
Data Science for Sustainability and Climate Change
Predicting Climate Patterns with Data
As the world grapples with the challenges of climate change, data science is emerging as a powerful tool to predict and mitigate its effects. By analyzing historical climate data, machine learning algorithms can forecast future trends in temperature, precipitation, and extreme weather events. These predictions can help governments and organizations prepare for and adapt to changing environmental conditions.
In addition, data science is being used to track carbon emissions, deforestation rates, and other environmental metrics in real-time. This data-driven approach allows policymakers and scientists to take informed actions to protect ecosystems and promote sustainability on a global scale.
How Data Science Can Support Sustainable Development Goals
The United Nations’ Sustainable Development Goals (SDGs) provide a blueprint for creating a more sustainable and equitable world. Data science plays a crucial role in achieving these goals by helping to measure progress, identify challenges, and optimize solutions. For example, data-driven models can help optimize energy usage, reduce waste in supply chains, and promote sustainable agricultural practices.
As data collection and processing technologies improve, data scientists will be able to tackle complex environmental issues with greater precision and scale. This shift towards data-driven sustainability efforts is essential for addressing the global challenges of climate change, resource scarcity, and environmental degradation.
Career Opportunities in Data Science
New Roles in Data Science: Data Ethicist, AI Trainer, etc.
As data science evolves, so do the roles within the field. The demand for specialized skills has led to the creation of new job titles that didn’t exist a few years ago. For example, Data Ethicists are now being employed to ensure that data practices align with ethical standards and regulations, particularly around privacy and fairness. AI Trainers are another emerging role, responsible for training AI systems using large datasets and ensuring that machine learning models are accurate and unbiased.
The future will see the rise of more niche roles as data science applications expand into different industries. Jobs like Machine Learning Engineer, Data Governance Specialist, and Quantitative Data Analyst will become increasingly important as the field diversifies.
Upskilling and Learning Paths for Aspiring Data Scientists
For those looking to enter or advance in the field of data science, the need for upskilling has never been more critical. Data science is a multidisciplinary field, requiring knowledge in statistics, machine learning, programming, and domain-specific expertise. Learning platforms like Coursera, edX, and Udemy offer a variety of courses tailored to these needs, making it easier for aspiring data scientists to acquire the necessary skills.
The rise of specialized certifications in areas like data engineering, machine learning, and AI ethics will further support professionals looking to deepen their expertise. As the demand for data science talent grows, continuous learning and professional development will remain key to staying competitive in this rapidly evolving field.
The Importance of Data Collaboration
Open Data Platforms and Their Future
The future of data science is increasingly collaborative, with open data platforms playing a pivotal role in innovation. Open data initiatives allow for the sharing of valuable datasets across industries, governments, and academic institutions. These platforms not only foster transparency but also create opportunities for cross-disciplinary research and development.
Projects like Kaggle, OpenAI, and government-led open data initiatives are just a few examples of how open data platforms are being used to solve complex global challenges. In the future, we can expect these platforms to grow, with more organizations contributing to shared databases. This openness will allow data scientists worldwide to collaborate, leading to faster advancements in AI, machine learning, and analytics.
How Data Sharing Can Foster Innovation
Data sharing between companies, research institutions, and governments can lead to breakthrough innovations, as pooling resources allows for larger and more diverse datasets. When diverse datasets are shared, data scientists can generate more accurate models, uncover hidden insights, and make discoveries that would be impossible with limited data.
One of the most notable benefits of data sharing is the acceleration of scientific research. In healthcare, for example, collaborative data initiatives can speed up drug discovery and improve the understanding of diseases. In the coming years, data collaboration will become increasingly important, as industries recognize the value of shared knowledge and collective problem-solving.
The Future of Data Visualization
The Role of Augmented Reality (AR) in Data Visualization
Augmented Reality (AR) is expected to revolutionize how data is visualized and interpreted in the future. AR technology can overlay digital information onto the physical world, allowing users to interact with data in real-time and in a more immersive way. Data scientists will be able to create dynamic visualizations that users can explore in a 3D space, transforming how businesses analyze and make decisions from data.
For example, imagine a retailer using AR to visualize sales data within a virtual store layout. By interacting with this data in real-time, the retailer could make informed decisions on product placement, inventory management, and customer experience optimization. As AR technology continues to improve, its integration with data science will create new opportunities for interactive and engaging data visualizations.
Advancements in Interactive Data Dashboards
Interactive data dashboards have become a cornerstone of data visualization, allowing users to explore data through filters, charts, and graphs. Tools like Tableau, Power BI, and Google Data Studio have made it easier for businesses to interact with data, enabling users to discover insights quickly and intuitively.
In the future, interactive dashboards will become even more sophisticated, incorporating AI-driven analytics and predictive models that allow users to forecast trends and make data-driven decisions with greater accuracy. Additionally, advancements in natural language processing (NLP) will allow users to interact with dashboards through voice commands, further simplifying the analysis process and making data more accessible to non-technical users.
The Globalization of Data Science
Data Science Trends in Emerging Markets
Data science is no longer confined to developed nations. Emerging markets are quickly becoming key players in the global data science ecosystem. Countries like India, Brazil, and China are experiencing rapid growth in their tech sectors, leading to increased investment in data science capabilities. These markets are home to vast populations and unique datasets, providing opportunities for local innovation and global collaboration.
As more industries in emerging markets adopt data science to solve local challenges—such as optimizing agricultural practices, improving healthcare infrastructure, or enhancing financial inclusion—data scientists in these regions will contribute to the global knowledge base. This will create a more interconnected world of data science, where solutions are shared across borders to address both regional and global problems.
Cross-Cultural Impacts of Global Data Sharing
The globalization of data science also brings a unique set of challenges and opportunities related to cross-cultural impacts. As data from different parts of the world becomes more interconnected, it’s crucial to consider the cultural contexts behind the data. For example, consumer behaviors, health trends, and economic activities can vary widely from one region to another, and these differences must be taken into account when building global models.
Additionally, global data sharing requires a thoughtful approach to ethics and privacy, particularly in countries with different regulations and attitudes towards data protection. In the future, data scientists will need to collaborate across borders, respecting cultural differences and navigating the complexities of international data governance.
Challenges in the Future of Data Science
Managing Data Complexity
As data science continues to evolve, managing the increasing complexity of data will be one of the biggest challenges for professionals in the field. Data is not only growing in volume but also in variety, with structured, semi-structured, and unstructured data coming from a wide range of sources such as IoT devices, social media, and enterprise systems. Handling this complexity requires sophisticated tools, scalable storage solutions, and efficient processing techniques.
Moreover, ensuring the quality and reliability of such complex data sets is crucial for generating accurate insights. Data scientists will need to focus more on data governance and quality management, utilizing techniques such as data lineage tracking, anomaly detection, and automated data cleaning tools.
Data Security in a Globalized World
With the globalization of data, security concerns are becoming more pronounced. As organizations collect and store increasing amounts of personal and sensitive information, the risk of data breaches and cyberattacks grows. Protecting this data, especially when it’s shared across borders, poses a significant challenge.
In the future, data security will become even more critical as stricter regulations come into play, and organizations face higher scrutiny over how they manage and protect their data. Technologies such as encryption, blockchain, and secure multi-party computation will play a key role in ensuring that data remains secure while still enabling collaboration and innovation.
Conclusion
The future of data science is full of exciting opportunities and challenges. As data continues to grow exponentially, emerging technologies like AI, quantum computing, and blockchain will revolutionize how data is processed, analyzed, and secured. Automation will play a major role in simplifying data science workflows, while ethical considerations around privacy, bias, and data security will become increasingly important.
Data science’s impact will extend across industries, from healthcare and finance to sustainability and global collaboration, driving innovation and solving some of the world’s most pressing problems. The key to success in this evolving field will be the ability to adapt, upskill, and embrace new technologies while maintaining a focus on responsible and ethical practices. Ultimately, data science will continue to shape the future, helping organizations and societies make more informed decisions and achieve greater efficiency and impact.
FAQs
-
What is the role of AI in the future of data science? AI is expected to play a crucial role by automating tasks such as data preprocessing, model building, and decision-making. AI-driven tools will make data science more accessible and efficient, allowing non-experts to benefit from its insights.
-
How will quantum computing impact data science? Quantum computing will revolutionize data science by enabling faster and more complex data analysis. Quantum algorithms will allow for more efficient model building, solving problems that traditional computers struggle with.
-
What ethical concerns exist in data science? Key ethical concerns include data privacy, the risk of biased algorithms, and the transparency of AI models. Addressing these issues is essential for ensuring that data science is used responsibly.
-
How can data science help combat climate change? Data science can help predict climate patterns, track environmental data, and develop sustainable solutions by analyzing large datasets related to weather, carbon emissions, and resource use.
-
What skills are essential for future data scientists? Aspiring data scientists should focus on learning machine learning, programming (Python, R), statistical analysis, data visualization, and data engineering, while also keeping up with emerging technologies like AI and quantum computing.